๐โโ๏ธ ํด๋น ๊ธ์ Tensorflow๋ฅผ ์ฌ์ฉํ๊ธฐ์ ํ๊ฒฝ์ด ๊ตฌ์ถ๋์ด์ผ ํฉ๋๋ค.
Anaconda3 + tensorflow ํค์๋๋ก ๊ตฌ๊ธ๋งํด์ ๋์ค๋ ๋ธ๋ก๊ทธ๋ค์ ์ฐธ๊ณ ๋ฐ๋๋๋ค. :)
00. ๋ชฉํ
๋๋ผ ์ ๋ณด ์ด๋ฏธ์ง๋ฅผ ๋ฃ์์ ๋, ์ํ(ํด๋น ๊ธ์์๋ ์์ ๋ง ๊ตฌ๋ถ)๋ฅผ ๊ตฌ๋ถํ๋ ๋ชจ๋ธ์ ๋ง๋ญ๋๋ค.
โข Input : ์ ๋ณด ์ด๋ฏธ์ง
โข Output : ์์
์๋๋ ํด๋น ๊ธ์์ ์ฌ์ฉํ ๋ฐ์ดํฐ์ ์ํ์ ๋๋ค. ๊ฐ ๋ง๋์ ๋ํ ์ด๋ฏธ์ง์ ๋ผ๋ฒจ ๋ฐ์ดํฐ๋ก ์ด๋ฃจ์ด์ ธ ์์ต๋๋ค.
๋๋ผ ๋ฐ์ดํฐ ์
๋ฐ์ดํฐ์ ์ ์๋์ ๊ฐ์ต๋๋ค.
ํนํ, ๋ผ๋ฒจ์ Alfaro๊ฐ ๋จ์ผ์ํฅ ์์ ์ ์ข์์ ์ฐ๋ก ์ฝ๋ 1์ฐจ์ ์ํ์ค๋ก ๋ํ๋ด๊ธฐ ์ํด ์ ์ํ ํํ์ ๋๋ค.
์ด ์ธ์ฝ๋ฉ์ ๊ฐ ์ฐจ๋ก๋๋ก ๋ํ๋๋ note์ symbol ์ฌ์ด์ '+' ๊ธฐํธ๋ฅผ ์ถ๊ฐํ๊ณ , ์ฝ๋์ ๊ฐ๋ณ ์ํ๋ฅผ ์๋์์ ์ ์์๋๋ก ๋์ดํฉ๋๋ค. ๊ทธ๋ฆฌ๊ณ ์ํ๊ฐ ๋์์ ๋์จ ๊ฒฝ์ฐ๋ '|' ๊ธฐํธ๋ฅผ ์ถ๊ฐํฉ๋๋ค.
ํด๋น ๊ธ์์๋ ์์ ๋ง์ ๊ตฌ๋ถํ๊ธฐ ๋๋ฌธ์ non-note ์์ ๊ธฐํธ(clefs, key signatures, time signatures, and barlines)์ ์ผํ๋ nonote๋ก ๊ตฌ๋ถํฉ๋๋ค.
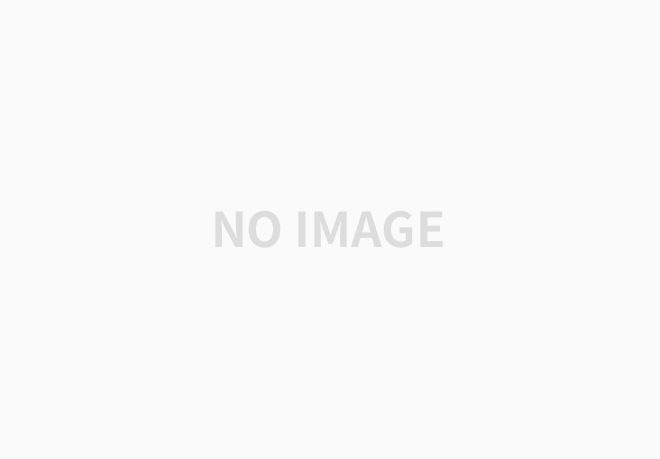
clef-percussion+note-F4_quarter|note-A5_quarter+note-C5_eighth|note-G5_eighth+note-G5_eighth+note-F4_eighth|note-G5_eighth+note-F4_eighth|note-G5_eighth+note-C5_eighth|note-G5_eighth+note-G5_eighth+barline
๋ผ๋ฒจ
(์ฐธ๊ณ ) ์์ ํ
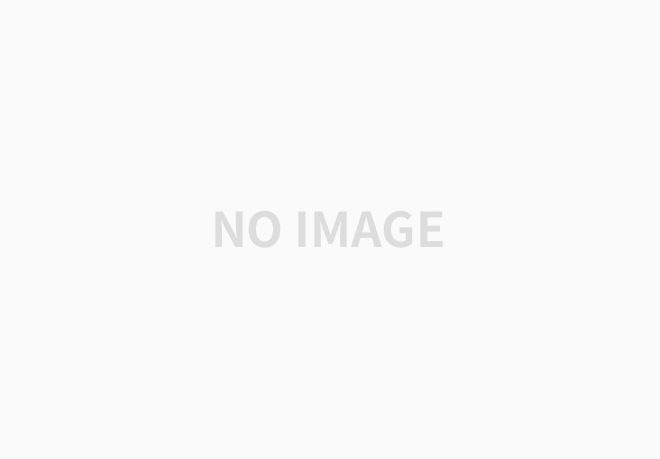
01. ๋ฐฐ๊ฒฝ
์ด๋ป๊ฒ ํ์ตํ๊ณ ์ํ๋ฅผ ๊ตฌ๋ถํ ์ ์๋์ง ์๋ฆฌ๋ฅผ ๊ฐ๋จํ๊ฒ ์ดํด๋ณด๊ฒ ์ต๋๋ค.
ํ์ต์๋ 3๊ฐ์ง ์๊ณ ๋ฆฌ์ฆ์ด ์ฌ์ฉ๋ฉ๋๋ค.
- CNN (Convolution Neural Network)
- RNN (Recurrent Neural Network)
- CTC Algorithm (Connectionist Temporal Classification)
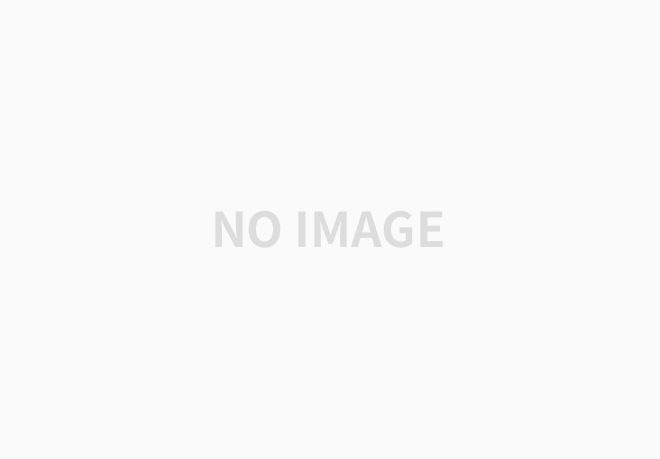
CNN์ ํตํด ์ด๋ฏธ์ง๋ก๋ถํฐ Feature Sequence๋ฅผ ์ถ์ถํฉ๋๋ค.
์ถ์ถํ Feature Sequence๋ค์ RNN์ Input์ผ๋ก ํ์ฌ ์ด๋ฏธ์ง์ Text Sequence๋ฅผ ์์ธกํฉ๋๋ค.
์ด๋ ๊ฒ CNN + RNN ์ผ๋ก ์ด๋ฃจ์ด์ง ๋ชจ๋ธ์ CRNN์ด๋ผ๊ณ ํฉ๋๋ค.
๊ทธ๋ผ Sequence Modeling์์ CRNN์ ์ฌ์ฉํ๋ ์ด์ ๋ ๋ฌด์์ผ๊น์?
CNN์ ์ ์ฒด ์ด๋ฏธ์ง์์ ํน์ ๋ถ๋ถ๋ง ๋ฐ์ํ๊ธฐ ๋๋ฌธ์ ์ ์ฒด ์ด๋ฏธ์ง์ ๋ํ ์ ๋ณด๋ฅผ ๋ด์ ์ ์๋ค๋ ํ๊ณ๊ฐ ์์ต๋๋ค.
์ด๋ฅผ ๋ณด์ํ๊ธฐ ์ํด ์ ๋ ฅ๊ณผ ์ถ๋ ฅ์ ์ํ์ค ๋จ์๋ก ์ฒ๋ฆฌํ๋ RNN์ ์ฌ์ฉํ์ฌ ์ ๋ณด๋ฅผ ์ข ํฉํด์ ๋ฌธ์๋ฅผ ์์ธกํ ์ ์๋๋ก ํ๋ ๊ฒ์ ๋๋ค.
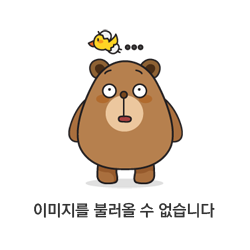
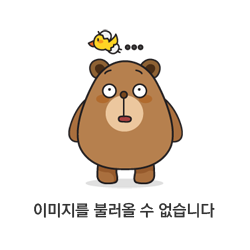
CTC๋ ์์ฑ ์ธ์๊ณผ ๋ฌธ์ ์ธ์์์ ์ฌ์ฉ๋๋ ์๊ณ ๋ฆฌ์ฆ์ ๋๋ค.
์์ฑ ํน์ ์ด๋ฏธ์ง๋ก๋ถํฐ ์ด๋์๋ถํฐ ์ด๋๊น์ง๊ฐ ํ ๋ฌธ์์ ํด๋นํ๋์ง ํ์ ํ๋ ๊ฒ์ด ์ด๋ ต๊ธฐ ๋๋ฌธ์ ๊ด๊ณ ์ ๋ ฌ์ ์ํด ์ฌ์ฉ๋ฉ๋๋ค.
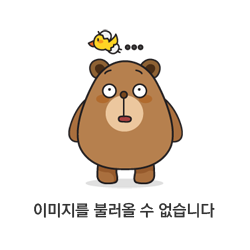
CTC๋ ์ด๋ฏธ์ง์ ๋ํด ์์๋ก ๋ถํ ๋ ๊ฐ ์์ญ๋ง๋ค์ ํน์ง์ ๋ํด ํ๋ฅ ์ ์ผ๋ก ์์ธกํ๊ฒ ๋ฉ๋๋ค.
P(Y|X) ์ฆ, ์ฃผ์ด์ง X์ ๋ํด์ Y์ผ ์กฐ๊ฑด๋ถ ํ๋ฅ ์ ๊ณ์ฐํด ์ฃผ๊ฒ ๋ฉ๋๋ค.
์ ๊ทธ๋ฆผ์์ ฯต์ Blank Token์ด๋ผ๊ณ ๋ถ๋ฅด๋๋ฐ, ๋ฌธ์ ์ด๋ฏธ์ง๊ฐ ์๋ ๋ถ๋ถ์ ๋น์นธ(Blank)์ผ๋ก ์ฒ๋ฆฌํ๊ณ
๊ฐ ๋จ๊ณ๋ณ ์์ธก๋ ์ค๋ณต ๋ฌธ์๋ค์ ํฉ์ณ์ ์ต์ข
๋ฌธ์๋ฅผ ์ป๊ฒ ๋ฉ๋๋ค.
02. Library
ํ์ํ ๋ผ์ด๋ธ๋ฌ๋ฆฌ๋ฅผ ๋ถ๋ฌ์ค๊ฒ ์ต๋๋ค.
import glob
import numpy as np
import matplotlib.pyplot as plt
import tensorflow as tf
from tensorflow import keras
from tensorflow.keras import layers
from sklearn.model_selection import train_test_split
03. Data Load
์ด๋ฏธ์ง์ Label์ ๋ด์ ๋ฆฌ์คํธ๋ฅผ ์์ฑํด์ฃผ๊ฒ ์ต๋๋ค.
x_dataset_path=f"{dataset_path}/measure/"
x_all_dataset_path = glob.glob(f"{x_dataset_path}/*")
x_file_list = [file for file in x_all_dataset_path if file.endswith(f".png")]
x_file_list.sort()
y_dataset_path=f"{dataset_path}/annotation/"
y_all_dataset_path = glob.glob(f"{y_dataset_path}/*")
y_file_list = [file for file in y_all_dataset_path if file.endswith(f".txt")]
y_file_list.sort()
images = x_file_list
labels = y_file_list
print("์ด ์ด๋ฏธ์ง ๊ฐ์: ", len(images))
print("์ด ๋ผ๋ฒจ ๊ฐ์: ", len(labels))
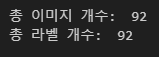
๋ฐฐ์น ์ฌ์ด์ฆ, ์ด๋ฏธ์ง ํฌ๊ธฐ ๋ฑ๋ ์ง์ ํด์ค๋๋ค.
# ๋ฐฐ์น ์ฌ์ด์ฆ ์ง์
batch_size = 16
# ์ด๋ฏธ์ง ํฌ๊ธฐ ์ง์
img_width = 256
img_height = 128
# ์ ์ผ ๊ธด ๋ผ๋ฒจ ๊ธธ์ด
max_length = 24
04. Data Pre-Processing
๋ฌธ์๋ฅผ ์ซ์๋ก encoding ํ๊ณ , ์ซ์๋ฅผ ๋ฌธ์๋ก decoding ํ๊ธฐ ์ํ char_to_num๊ณผ num_to_char๋ฅผ ๋ง๋ค์ด์ฃผ๊ฒ ์ต๋๋ค.
์ฐ์ Pitch(์์ )์ ๋ํด ๊ตฌ๋ถํ๋ ๋ชจ๋ธ์ ์ํด ํ์ํ vocabulary๋ฅผ ์๋์ ๊ฐ์ด ์ ์ํ๊ฒ ์ต๋๋ค.
char_to_int_mapping = [
"|", #1
"nonote",#2
"note-D4",#3
"note-E4",#4
"note-F4",#5
"note-G4",#6
"note-A4",#7
"note-B4",#8
"note-C5",#9
"note-D5",#10
"note-E5",#11
"note-F5",#12
"note-G5",#13
"note-A5",#14
"note-B5",#15
]
# ๋ฌธ์๋ฅผ ์ซ์๋ก ๋ณํ
char_to_num = layers.StringLookup(
vocabulary=list(char_to_int_mapping), mask_token=None
)
# ์ซ์๋ฅผ ๋ฌธ์๋ก ๋ณํ
num_to_char = layers.StringLookup(
vocabulary=char_to_num.get_vocabulary(), mask_token=None, invert=True
)
char_to_num.get_vocabulary() ์ผ๋ก ๋ค์๊ณผ ๊ฐ์ด ์ง์ ๋ vocabulary๋ฅผ ํ์ธํ ์ ์์ต๋๋ค.

ํ์ฌ ๋ผ๋ฒจ์ ์ํ, ์ผํ, ๋ง๋์ ๋ฑ ๋ชจ๋ ๊ฒ ๋ค ํฌํจ๋์ด ์๊ธฐ ๋๋ฌธ์ ์ด์ค์์ ์ํ ์ ๋ณด๋ง ๊ฐ์ ธ์ vocabulary์ ๋งคํ๋๋๋ก ์ฒ๋ฆฌํ๊ฒ ์ต๋๋ค.
# ๊ฐ token์ ๋ง๋ string list๋ก ๋ง๋ค๊ธฐ
def map_pitch(note):
pitch_mapping = {
"note-D4": 1,
"note-E4": 2,
"note-F4": 3,
"note-G4": 4,
"note-A4": 5,
"note-B4": 6,
"note-C5": 7,
"note-D5": 8,
"note-E5": 9,
"note-F5": 10,
"note-G5": 11,
"note-A5": 12,
"note-B5": 13,
}
return "nonote" if note not in pitch_mapping else note
def map_rhythm(note):
duration_mapping = {
"[PAD]":0,
"+": 1,
"|": 2,
"barline": 3,
"clef-percussion": 4,
"note-eighth": 5,
"note-eighth.": 6,
"note-half": 7,
"note-half.": 8,
"note-quarter": 9,
"note-quarter.": 10,
"note-16th": 11,
"note-16th.": 12,
"note-whole": 13,
"note-whole.": 14,
"rest_eighth": 15,
"rest-eighth.": 16,
"rest_half": 17,
"rest_half.": 18,
"rest_quarter": 19,
"rest_quarter.": 20,
"rest_16th": 21,
"rest_16th.": 22,
"rest_whole": 23,
"rest_whole.": 24,
"timeSignature-4/4": 25
}
return note if note in duration_mapping else "<unk>"
def map_lift(note):
lift_mapping = {
"lift_null" : 1,
"lift_##" : 2,
"lift_#" : 3,
"lift_bb" : 4,
"lift_b" : 5,
"lift_N" : 6
}
return "nonote" if note not in lift_mapping else note
def symbol2pitch_rhythm_lift(symbol_lift, symbol_pitch, symbol_rhythm):
return map_lift(symbol_lift), map_pitch(symbol_pitch), map_rhythm(symbol_rhythm)
def note2pitch_rhythm_lift(note):
# note-G#3_eighth
note_split = note.split("_") # (note-G#3) (eighth)
note_pitch_lift = note_split[:1][0]
note_rhythm = note_split[1:][0]
rhythm=f"note-{note_rhythm}"
note_note, pitch_lift = note_pitch_lift.split("-") # (note) (G#3)
if len(pitch_lift)>2:
pitch = f"note-{pitch_lift[0]+pitch_lift[-1]}" # (G3)
lift = f"lift_{pitch_lift[1:-1]}"
else:
pitch = f"note-{pitch_lift}"
lift = f"lift_null"
return symbol2pitch_rhythm_lift(lift, pitch, rhythm)
def rest2pitch_rhythm_lift(rest):
# rest-quarter
return symbol2pitch_rhythm_lift("nonote", "nonote", rest)
def map_pitch2isnote(pitch_note):
group_notes = []
note_split = pitch_note.split("+")
for note_s in note_split:
if "nonote" in note_s:
group_notes.append("nonote")
elif "note-" in note_s:
group_notes.append("note")
return "+".join(group_notes)
def map_notes2pitch_rhythm_lift_note(note_list):
result_lift=[]
result_pitch=[]
result_rhythm=[]
result_note=[]
for notes in note_list:
group_lift = []
group_pitch = []
group_rhythm = []
group_notes_token_len=0
# ์ฐ์ +๋ก ๋๋๊ณ , ์์ | ์๋ ์ง ํ์ธํด์ ๋จผ์ ๋ถ์ด๊ธฐ
# note-G#3_eighth + note-G3_eighth + note-G#3_eighth|note-G#3_eighth + rest-quarter
note_split = notes.split("+")
for note_s in note_split:
if "|" in note_s:
mapped_lift_chord = []
mapped_pitch_chord = []
mapped_rhythm_chord = []
# note-G#3_eighth|note-G#3_eighth
note_split_chord = note_s.split("|") # (note-G#3_eighth) (note-G#3_eighth)
for idx, note_s_c in enumerate(note_split_chord):
chord_lift, chord_pitch, chord_rhythm = note2pitch_rhythm_lift(note_s_c)
mapped_lift_chord.append(chord_lift)
mapped_pitch_chord.append(chord_pitch)
mapped_rhythm_chord.append(chord_rhythm)
# --> '|' ๋ token์ด๊ธฐ ๋๋ฌธ์ lift, pitch์ nonote ์ถ๊ฐํด์ฃผ๊ธฐ
if idx != len(note_split_chord)-1:
mapped_lift_chord.append("nonote")
# mapped_pitch_chord.append("nonote")
group_lift.append(" ".join(mapped_lift_chord))
group_pitch.append(" | ".join(mapped_pitch_chord))
group_rhythm.append(" | ".join(mapped_rhythm_chord))
# --> '|' ๋ token์ด๊ธฐ ๋๋ฌธ์ ์ถ๊ฐ๋ token ๊ฐ์ ๋ํ๊ธฐ
# ๋์์ ์น ๊ฑธ ํ๋์ string์ผ๋ก ํด๋ฒ๋ฆฌ๋ ๊ฑฐ๋๊น ์ฃผ์ํ๊ธฐ
group_notes_token_len+=len(note_split_chord) + len(note_split_chord)-1
elif "note" in note_s:
if "_" in note_s:
# note-G#3_eighth
note2lift, note2pitch, note2rhythm = note2pitch_rhythm_lift(note_s)
group_lift.append(note2lift)
group_pitch.append(note2pitch)
group_rhythm.append(note2rhythm)
group_notes_token_len+=1
elif "rest" in note_s:
if "_" in note_s:
# rest_quarter
rest2lift, rest2pitch, rest2rhythm =rest2pitch_rhythm_lift(note_s)
group_lift.append(rest2lift)
group_pitch.append(rest2pitch)
group_rhythm.append(rest2rhythm)
group_notes_token_len+=1
else:
# clef-F4+keySignature-AM+timeSignature-12/8
symbol2lift, symbol2pitch, symbol2rhythm = symbol2pitch_rhythm_lift("nonote", "nonote", note_s)
group_lift.append(symbol2lift)
group_pitch.append(symbol2pitch)
group_rhythm.append(symbol2rhythm)
group_notes_token_len+=1
toks_len= group_notes_token_len
# lift, pitch
emb_lift= " ".join(group_lift)
emb_pitch= " ".join(group_pitch)
# rhythm
emb_rhythm= " ".join(group_rhythm)
# ๋ค์ ๋จ์ ๊ฑด ํจ๋ฉ
if toks_len < max_length :
for _ in range(max_length - toks_len ):
emb_lift+=" [PAD]"
emb_pitch+=" [PAD]"
emb_rhythm+=" [PAD]"
result_lift.append(emb_lift)
result_pitch.append(emb_pitch)
result_rhythm.append(emb_rhythm)
result_note.append(map_pitch2isnote(emb_pitch))
return result_lift, result_pitch, result_rhythm, result_note
def read_txt_file(file_path):
# ํ
์คํธ ํ์ผ์ ์ฝ์ด์ ๋ด์ฉ์ ๋ฆฌ์คํธ๋ก ๋ฐํ
with open(file_path, 'r', encoding='utf-8') as file:
content = file.readlines()
# ๊ฐ ์ค์ ๊ฐํ ๋ฌธ์ ์ ๊ฑฐ
content = [line.strip() for line in content]
return content[0]
contents = []
# ๊ฐ ํ์ผ์ ์ฝ์ด์ ๋ด์ฉ์ ๋ฆฌ์คํธ์ ์ถ๊ฐ
for annotation_path in labels:
content = read_txt_file(annotation_path)
# ์ฌ์ด์ฌ์ด์ + ๋ก ์ฐ๊ฒฐํด์ฃผ๊ธฐ
content=content.replace(" ","+")
content=content.replace("\t","+")
contents.append(content)
result_lift, result_pitch, result_rhythm, result_note = map_notes2pitch_rhythm_lift_note(contents)
labels=result_pitch
์ฒ๋ฆฌ๋ ๊ฑธ ํ์ธํด๋ณด๊ฒ ์ต๋๋ค.
print(contents[0])
print(labels[0])
print(char_to_num(tf.strings.split(labels[0])))

sklearn์ train_test_split()์ ์ด์ฉํด Data๋ฅผ Train Set๊ณผ Validation Set์ผ๋ก ๋๋์ด ๊ฐ ๋ณ์์ ์ ์ฅํฉ๋๋ค.
์ด Dataset์์ 90%๋ฅผ Train Set์ผ๋ก ์ฌ์ฉํ๊ณ 10%๋ฅผ Validation Set์ผ๋ก ์ง์ ํด ์ฃผ๊ธฐ ์ํด test_size๋ฅผ 0.1๋ก ์ค์ ํ์ต๋๋ค.
x_train, x_valid, y_train, y_valid = train_test_split(np.array(images), np.array(labels), test_size=0.1)
๋ง์ง๋ง์ผ๋ก Dataset ์์ฑ ์ ์ ์ฉ๋ encode_single_sample() ํจ์๋ฅผ ์ง์ ํด์ค๋๋ค. ์ด ํจ์๋ฅผ ์ด์ฉํด ๋ฐ์ดํฐ๋ฅผ tensorflow์ ์ ํฉํ ํํ๋ก ๋ณํ์์ผ์ค ์ ์๋๋ก ํฉ๋๋ค.
์ด๋ฏธ์ง๋ ๋ฐ์ด๋๋ฆฌ ์ด๋ฏธ์ง๋ก ๋ณํ๋๊ณ , ์์์ ์ง์ ํ ํฌ๊ธฐ์ ๋ง๊ฒ resize๋ฉ๋๋ค. ์ดํ, ์ด๋ฏธ์ง๊ฐ ์๋ ๊ฐ๋ก๋ก ๊ธด ํํ์๋๋ฐ ์ฒซ ์ํ๋ถํฐ ์์ฐจ์ ์ผ๋ก ํด์ํ๊ธธ ์ํ๊ธฐ ๋๋ฌธ์ ์์์๋ถํฐ ์๋๋ก ์ด๋ฏธ์ง๋ฅผ ์ฝ์ ์ ์๋๋ก ์ด๋ฏธ์ง์ ๊ฐ๋ก ์ธ๋ก๋ฅผ ๋ณํํฉ๋๋ค.
๋ผ๋ฒจ์ ๊ฐ string๋ง๋ค splitํ์ฌ encoding๋๋๋ก ํฉ๋๋ค.
def encode_single_sample(img_path, label):
# 1. ์ด๋ฏธ์ง ๋ถ๋ฌ์ค๊ธฐ
img = tf.io.read_file(img_path)
# 2. ์ด๋ฏธ์ง๋ก ๋ณํํ๊ณ grayscale๋ก ๋ณํ
img = tf.io.decode_png(img, channels=1)
# 3. [0,255]์ ์ ์ ๋ฒ์๋ฅผ [0,1]์ ์ค์ ๋ฒ์๋ก ๋ณํ
img = tf.image.convert_image_dtype(img, tf.float32)
# 4. ์ด๋ฏธ์ง resize
img = tf.image.resize(img, [img_height, img_width])
# 5. ์ด๋ฏธ์ง์ ๊ฐ๋ก ์ธ๋ก ๋ณํ
img = tf.transpose(img, perm=[1, 0, 2])
# 6. ๋ผ๋ฒจ ๊ฐ์ ๋ฌธ์๋ฅผ ์ซ์๋ก ๋ณํ
label_r = char_to_num(tf.strings.split(label))
# 7. ๋์
๋๋ฆฌ ํํ๋ก return
return {"image": img, "label": label_r}
05. Dataset ๊ฐ์ฒด ์์ฑ
tf.data.Dataset์ ์ด์ฉํ์ฌ numpy array ํน์ tensor๋ก๋ถํฐ Dataset์ ๋ง๋ค์ด์ฃผ๊ฒ ์ต๋๋ค.
์์์ ์ ์ํ encode_single_sample ํจ์๋ฅผ ์ ์ํ์ฌ ์์์ ์ง์ ํ batch size๋ก train, validation Dataset์ ๋ง๋ค์ด์ฃผ๊ฒ ์ต๋๋ค.
train_dataset = tf.data.Dataset.from_tensor_slices((x_train, y_train))
train_dataset = (
train_dataset.map(
encode_single_sample, num_parallel_calls=tf.data.AUTOTUNE
)
.batch(batch_size)
.prefetch(buffer_size=tf.data.AUTOTUNE)
)
validation_dataset = tf.data.Dataset.from_tensor_slices((x_valid, y_valid))
validation_dataset = (
validation_dataset.map(
encode_single_sample, num_parallel_calls=tf.data.AUTOTUNE
)
.batch(batch_size)
.prefetch(buffer_size=tf.data.AUTOTUNE)
)
06. Data ์๊ฐํ
๋ง๋ค์ด์ง Dataset์ ์ด๋ฏธ์ง์ Label์ ํ์ธํด๋ณด๊ฒ ์ต๋๋ค.
์ด๋ฏธ์ง๋ ๊ฐ๋ก์ ์ธ๋ก๋ฅผ ๋ณํํ์๋๋ฐ, ์๋ ์ฝ๋์์๋ imshow(img[:, :, 0].T์์ T๋ฅผ ์ฌ์ฉํด์ ์ด๋ฏธ์ง๋ฅผ ๋ค์ Transpose ํ์ฌ ๋ณด๊ธฐ ์ฝ๊ฒ ์ถ๋ ฅํด ์ฃผ์์ต๋๋ค.
_, ax = plt.subplots(4, 1)
for batch in train_dataset.take(1):
images = batch["image"]
labels = batch["label"]
for i in range(4):
img = (images[i] * 255).numpy().astype("uint8")
label = tf.strings.join(num_to_char(labels[i]), separator=' ').numpy().decode("utf-8").replace('[UNK]', '')
print(labels[i])
ax[i].imshow(img[:, :, 0].T, cmap="gray")
ax[i].set_title(label)
ax[i].axis("off")
plt.show()
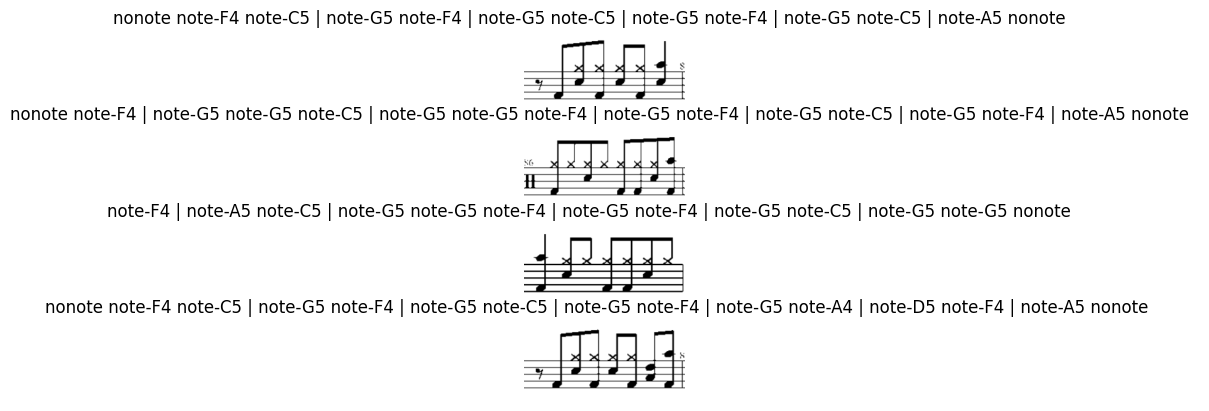
07. Model
CTC Loss๋ฅผ ๊ตฌํ๊ธฐ ์ํ CTC Layer ํด๋์ค๋ฅผ ๊ตฌํํ๊ฒ ์ต๋๋ค.
CTC Loss๋ keras.backend.ctc_batch_cost๋ฅผ ํตํด ๊ตฌํํ ์ ์์ต๋๋ค.
class CTCLayer(layers.Layer):
def __init__(self, name=None):
super().__init__(name=name)
self.loss_fn = keras.backend.ctc_batch_cost
def call(self, y_true, y_pred):
batch_len = tf.cast(tf.shape(y_true)[0], dtype="int64")
input_length = tf.cast(tf.shape(y_pred)[1], dtype="int64")
label_length = tf.cast(tf.shape(y_true)[1], dtype="int64")
input_length = input_length * tf.ones(shape=(batch_len, 1), dtype="int64")
label_length = label_length * tf.ones(shape=(batch_len, 1), dtype="int64")
loss = self.loss_fn(y_true, y_pred, input_length, label_length)
self.add_loss(loss)
return y_pred
CRNN ๋ชจ๋ธ์ ๊ตฌํํ๊ฒ ์ต๋๋ค. 2๊ฐ์ Convolution block๊ณผ 2๊ฐ์ LSTM ๋ชจ๋ธ์ด ๊ฒฐํฉํ ๋ชจ๋ธ์ ๋๋ค.
ํ์ต์ ์ฌ์ฉ๋๋ loss๋ ์์์ ์ง์ ํ CTC Layer์ loss๋ฅผ ์ด์ฉํด์ ํ์ตํ๋๋ก ์ค์ ํด ์ค๋๋ค.
def build_model():
# Inputs
input_img = layers.Input(
shape=(img_width, img_height, 1), name="image", dtype="float32"
)
labels = layers.Input(name="label", shape=(None,), dtype="float32")
# ์ฒซ๋ฒ์งธ convolution block
x = layers.Conv2D(
32,
(3, 3),
activation="relu",
kernel_initializer="he_normal",
padding="same",
name="Conv1",
)(input_img)
x = layers.MaxPooling2D((2, 2), name="pool1")(x)
# ๋๋ฒ์งธ convolution block
x = layers.Conv2D(
64,
(3, 3),
activation="relu",
kernel_initializer="he_normal",
padding="same",
name="Conv2",
)(x)
x = layers.MaxPooling2D((2, 2), name="pool2")(x)
# ์์ 2๊ฐ์ convolution block์์ maxpooling(2,2)์ ์ด 2๋ฒ ์ฌ์ฉ
# feature map์ ํฌ๊ธฐ๋ 1/4๋ก downsampling
# ๋ง์ง๋ง layer์ filter ์๋ 64๊ฐ ๋ค์ RNN์ ๋ฃ๊ธฐ ์ ์ reshape
new_shape = ((img_width // 4), (img_height // 4) * 64)
x = layers.Reshape(target_shape=new_shape, name="reshape")(x)
x = layers.Dense(64, activation="relu", name="dense1")(x)
x = layers.Dropout(0.2)(x)
# RNNs
x = layers.Bidirectional(layers.LSTM(128, return_sequences=True, dropout=0.25))(x)
x = layers.Bidirectional(layers.LSTM(64, return_sequences=True, dropout=0.25))(x)
# Output layer
x = layers.Dense(
len(char_to_num.get_vocabulary()) + 1, activation="softmax", name="dense2"
)(x)
# ctc loss
output = CTCLayer(name="ctc_loss")(labels, x)
# Model
model = keras.models.Model(
inputs=[input_img, labels], outputs=output, name="omr"
)
# Optimizer
opt = keras.optimizers.Adam()
model.compile(optimizer=opt)
return model
# Model
model = build_model()
model.summary()
08. Train
๊ทธ๋ผ ์ด์ epoch๋ฅผ 200์ผ๋ก ์ค์ ํ๊ณ early stopping์ patience๋ฅผ 10์ผ๋ก ์ง์ ํ์ฌ ํ์ต์ ํด๋ณด๋๋ก ํ๊ฒ ์ต๋๋ค.
epochs = 200
early_stopping_patience = 10
early_stopping = keras.callbacks.EarlyStopping(
monitor="val_loss", patience=early_stopping_patience, restore_best_weights=True
)
history = model.fit(
train_dataset,
validation_data=validation_dataset,
epochs=epochs,
callbacks=[early_stopping],
)
Epoch 1/200
6/6 [==============================] - 9s 339ms/step - loss: 99.2571 - val_loss: 62.9635
Epoch 2/200
6/6 [==============================] - 0s 49ms/step - loss: 58.3946 - val_loss: 49.3992
Epoch 3/200
6/6 [==============================] - 0s 42ms/step - loss: 51.5517 - val_loss: 46.9572
Epoch 4/200
6/6 [==============================] - 0s 42ms/step - loss: 49.1146 - val_loss: 45.2404
Epoch 5/200
6/6 [==============================] - 0s 40ms/step - loss: 47.8802 - val_loss: 43.4212
Epoch 6/200
6/6 [==============================] - 0s 41ms/step - loss: 45.6561 - val_loss: 41.9743
Epoch 7/200
6/6 [==============================] - 0s 40ms/step - loss: 44.0756 - val_loss: 41.5652
Epoch 8/200
6/6 [==============================] - 0s 39ms/step - loss: 43.6151 - val_loss: 38.8865
Epoch 9/200
6/6 [==============================] - 0s 39ms/step - loss: 41.6590 - val_loss: 39.4570
Epoch 10/200
6/6 [==============================] - 0s 40ms/step - loss: 41.6629 - val_loss: 38.0012
Epoch 11/200
6/6 [==============================] - 0s 40ms/step - loss: 40.3495 - val_loss: 37.4150
Epoch 12/200
6/6 [==============================] - 0s 38ms/step - loss: 39.3751 - val_loss: 38.2025
Epoch 13/200
6/6 [==============================] - 0s 39ms/step - loss: 38.5040 - val_loss: 37.1740
...
Epoch 171/200
6/6 [==============================] - 0s 39ms/step - loss: 2.8438 - val_loss: 2.2756
Epoch 172/200
6/6 [==============================] - 0s 40ms/step - loss: 2.7682 - val_loss: 2.1957
09. Predict
ํ์ต๋ ๋ชจ๋ธ๋ก Validation Data๋ฅผ ์์ ์ผ๋ก ์ถ๋ ฅํ๊ธฐ ์ํ ๋ชจ๋ธ์ ๋ง๋ค์ด์ค๋๋ค.
์ถ๋ ฅ๊ฐ์ Decodingํ๊ธฐ ์ํด decode_batch_predictions๋ผ๋ ํจ์๋ฅผ ์ง์ ํฉ๋๋ค.
# Prediction Model
prediction_model = keras.models.Model(
model.get_layer(name="image").input, model.get_layer(name="dense2").output
)
prediction_model.summary()
# Decoding
def decode_batch_predictions(pred):
input_len = np.ones(pred.shape[0]) * pred.shape[1]
results = keras.backend.ctc_decode(pred, input_length=input_len, greedy=True)[0][0][
:, :max_length
]
output_text = []
for res in results:
print(res)
res = tf.strings.join(num_to_char(res), separator=' ').numpy().decode("utf-8").replace('[UNK]', '')
output_text.append(res)
return output_text
10. ์์ธก ๊ฒฐ๊ณผ ํ์ธ
prediction_model์ validation_dataset ๋ฐฐ์น ํ ๊ฐ๋ฅผ ๋ฃ์ด ์๊ฐํํด๋ณด๊ฒ ์ต๋๋ค.
# validation dataset์์ ํ๋์ ๋ฐฐ์น๋ฅผ ์๊ฐํ
for batch in validation_dataset.take(1):
batch_images = batch["image"]
batch_labels = batch["label"]
preds = prediction_model.predict(batch_images)
pred_texts = decode_batch_predictions(preds)
orig_texts = []
for label in batch_labels:
label = tf.strings.join(num_to_char(label), separator=' ').numpy().decode("utf-8").replace('[UNK]', '')
orig_texts.append(label)
_, ax = plt.subplots(10, 1, figsize=(100, 50))
for i in range(len(pred_texts)):
img = (batch_images[i, :, :, 0] * 255).numpy().astype(np.uint8)
img = img.T
title = f"Prediction: {pred_texts[i]}"
ax[i].imshow(img, cmap="gray")
ax[i].set_title(title)
ax[i].axis("off")
plt.show()
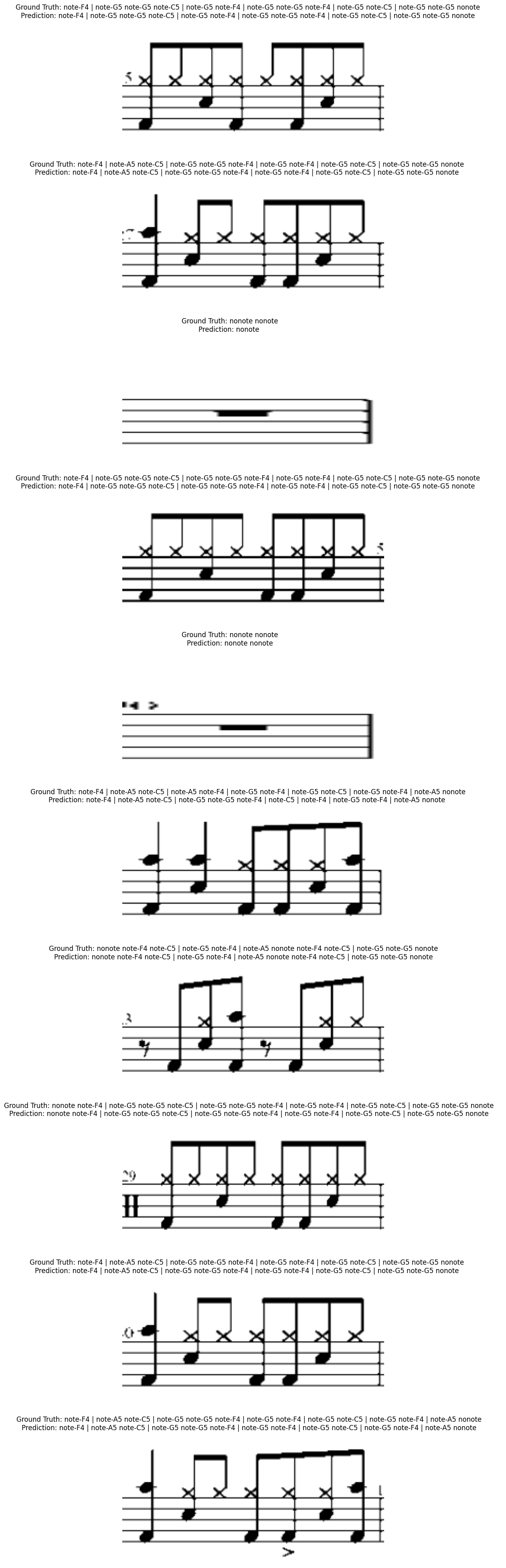
ํด๋น ๊ธ์ ์์ ๊ตฌ๋ถ์ ์ํ ๋ชจ๋ธ์ ๋๋ค.
์ผํ ๋ฐ ๋ง๋์ ๋ฑ์ ๊ตฌ๋ถํ๊ธฐ ์ํด์ ์ถ๊ฐ ๋ผ๋ฒจ๋ง ์์ ์ด ํ์ํฉ๋๋ค.
๋ฐ์ดํฐ์ ์ํ๋ 92๊ฐ๋ฐ์ ์์ด, ์ฑ๋ฅ์ด ์ข์ง ์์๋ฐ ๋ฐ์ดํฐ๋ฅผ ๋์ฉ๋์ ์์ฑํ์ฌ ํ์ตํ๋ฉด ๋ ์ข์ ์ฑ๋ฅ์ ๋ณด์ผ ๊ฒ์ผ๋ก ์์ํฉ๋๋ค.
๊ฐ์ฌํฉ๋๋ค.
์ฐธ๊ณ ๋ฌธํ
End-to-End Neural Optical Music Recognition of Monophonic Scores
Optical Music Recognition is a field of research that investigates how to computationally decode music notation from images. Despite the efforts made so far, there are hardly any complete solutions to the problem. In this work, we study the use of neural n
www.mdpi.com
'๐ป My Work > ๐ง AI' ์นดํ ๊ณ ๋ฆฌ์ ๋ค๋ฅธ ๊ธ
[๋ฅ๋ฌ๋] TensorFlow๋ฅผ ์ฌ์ฉํ ๋ฅ๋ฌ๋ CNN ๋๋ผ ์๋ฆฌ ๋ถ๋ฅ (0) | 2023.11.24 |
---|---|
[MiniHack] ํ๊ฒฝ ์ธํ (0) | 2023.01.04 |
[์ธ๊ณต์ง๋ฅ/ํผ๊ณต๋จธ์ ] 07-1. ์ธ๊ณต ์ ๊ฒฝ๋ง (3) (0) | 2023.01.02 |
[์ธ๊ณต์ง๋ฅ/ํผ๊ณต๋จธ์ ] 07-1. ์ธ๊ณต ์ ๊ฒฝ๋ง (2) (0) | 2023.01.01 |
[์ธ๊ณต์ง๋ฅ/ํผ๊ณต๋จธ์ ] 07-1. ์ธ๊ณต ์ ๊ฒฝ๋ง (1) (0) | 2022.12.17 |